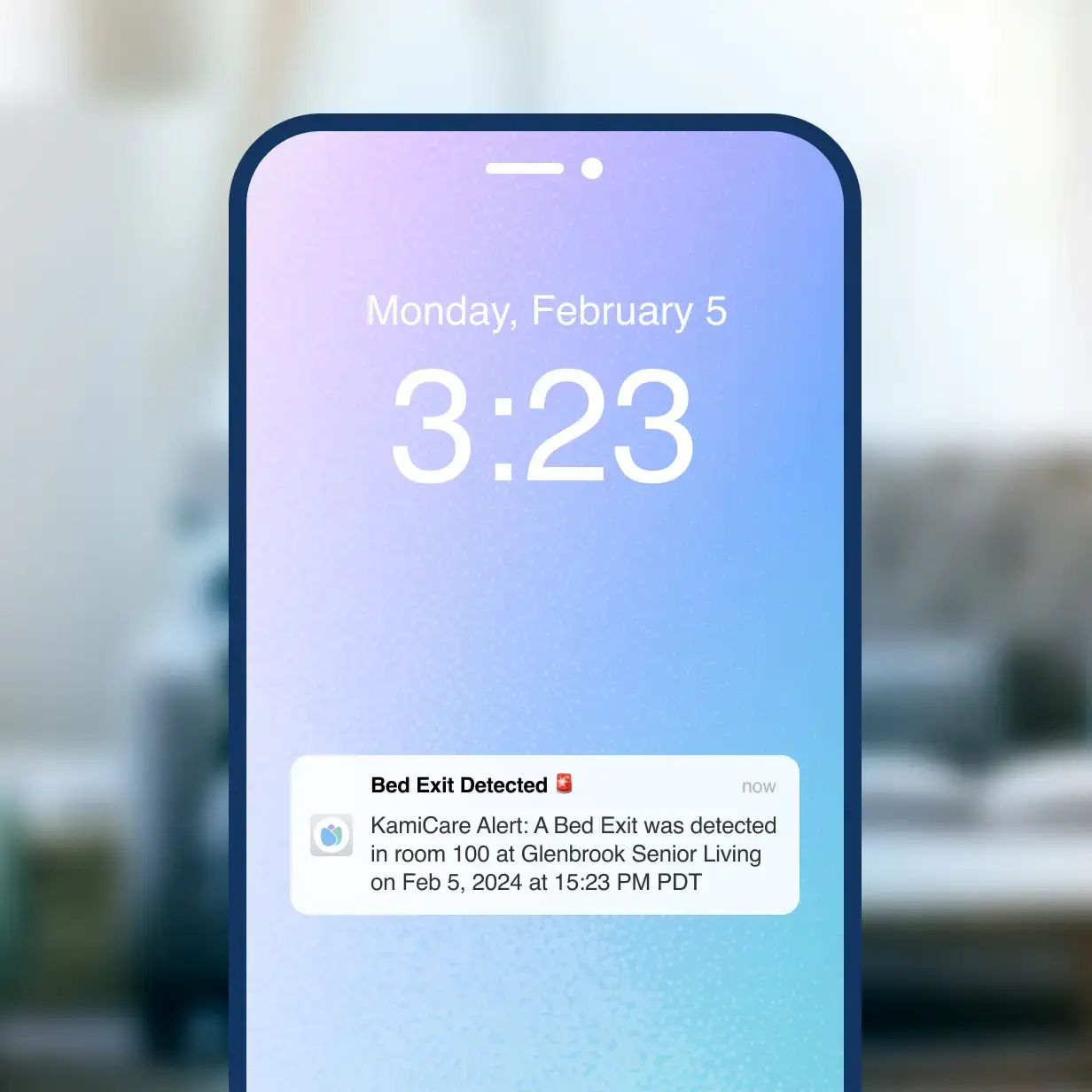
Step-by-Step Guide to set up Notifications in KamiCare
Effective notifications are key to ensuring rapid response in KamiCare. Whether you want alerts via phone call, ...
Read More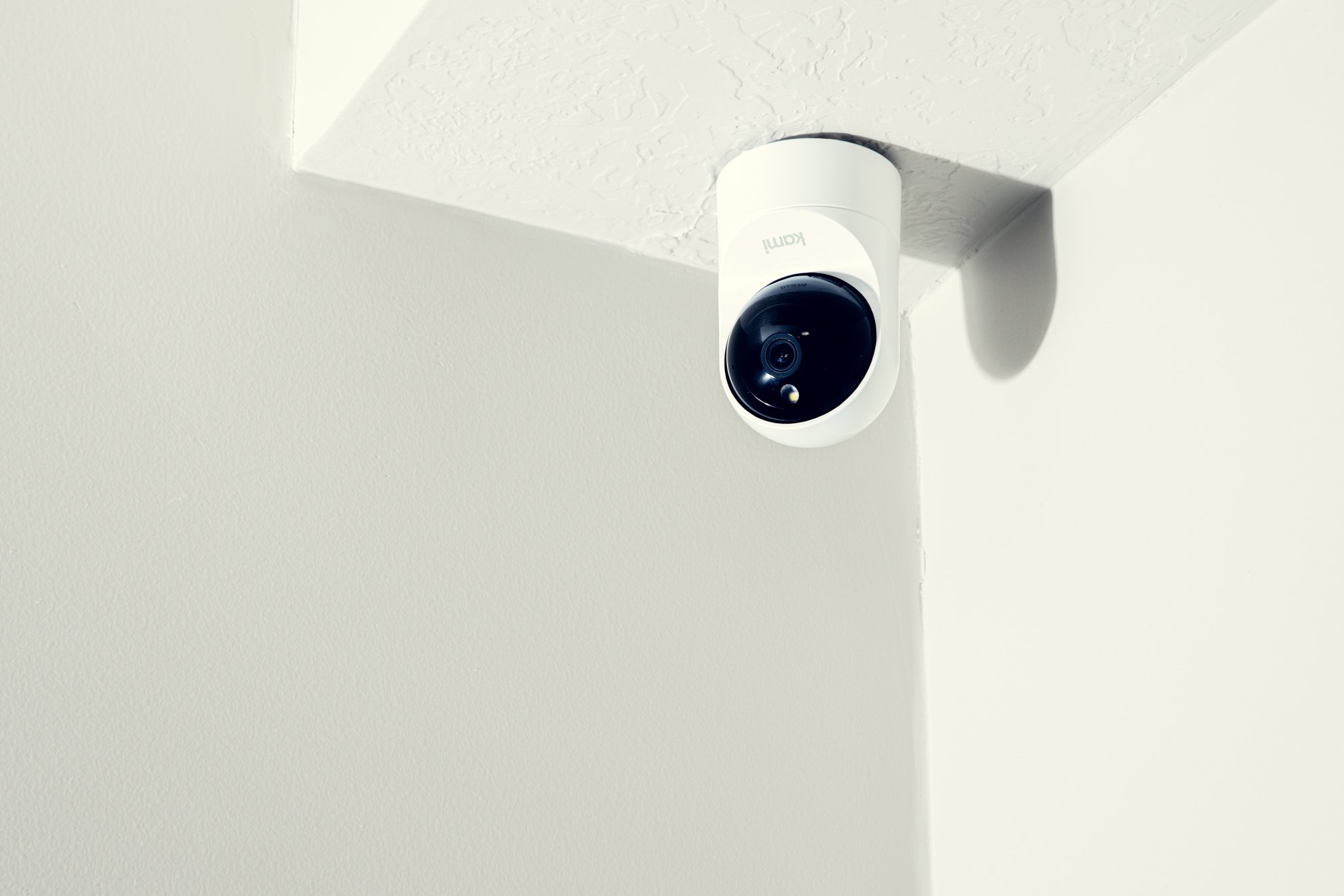
Step-by-Step Guide to Pairing Your KamiCare Camera
Pairing your KamiCare camera is a simple process that ensures you get accurate KamiCare alert capabilities. Follow ...
Read More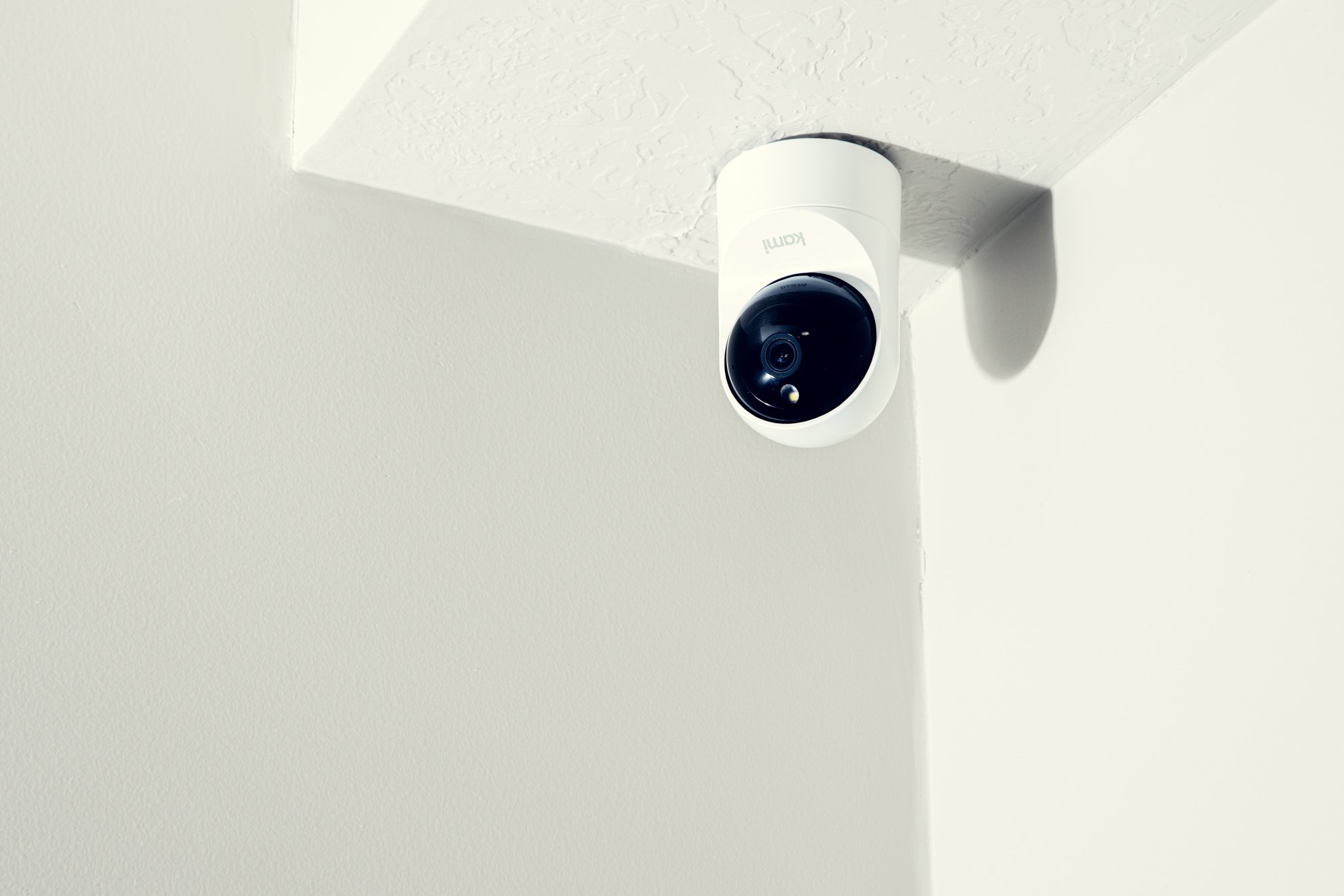
Step-by-Step Guide to Installing Your KamiCare Camera
Setting up your KamiCare camera is a straightforward process that ensures you get optimal fall-detection ...
Read More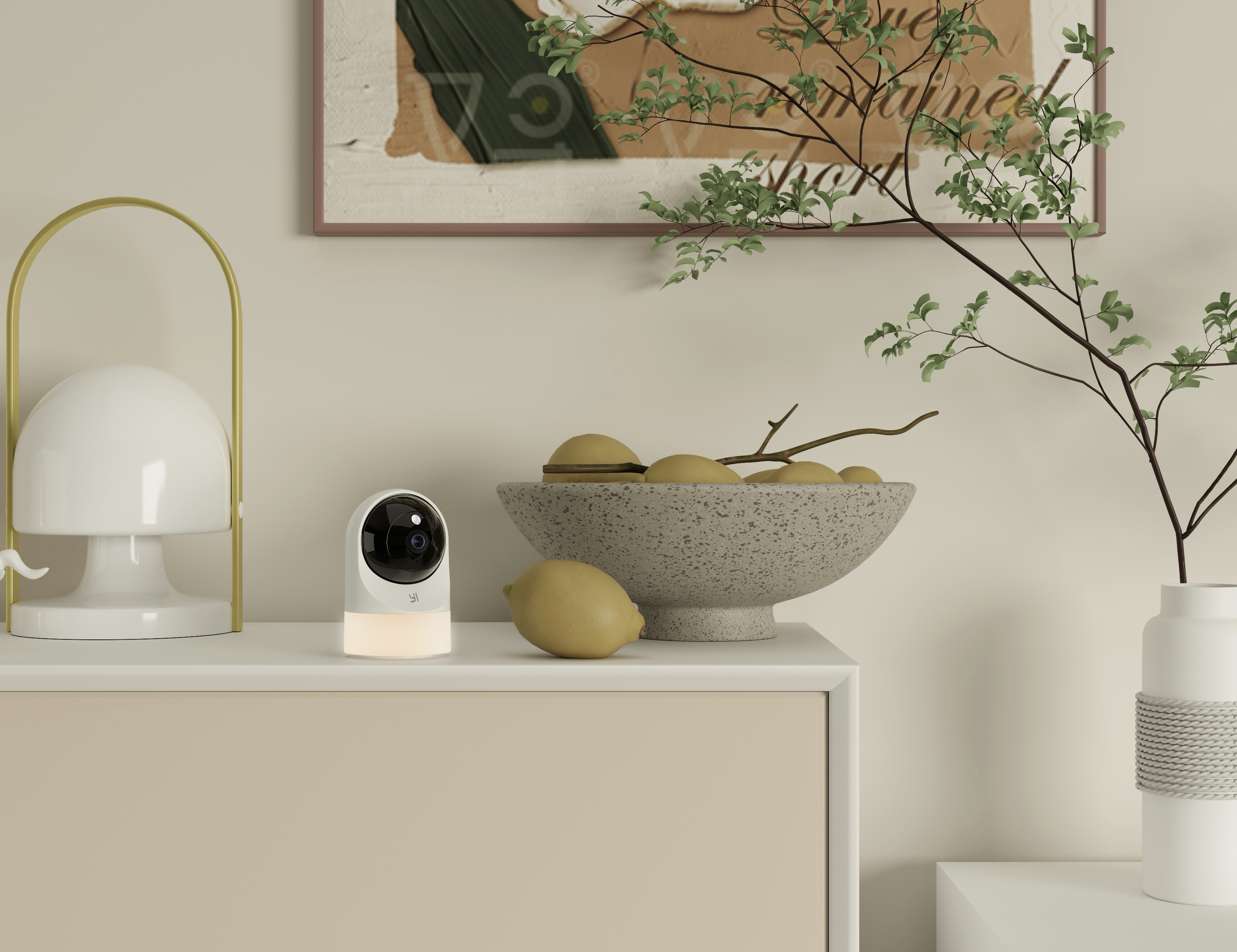
Kami Vision Displays Newest Innovations at CES 2025
Kami Vision participated in CES 2025 last week, presenting our latest advancements in Vision A.I. and wearable ...
Read More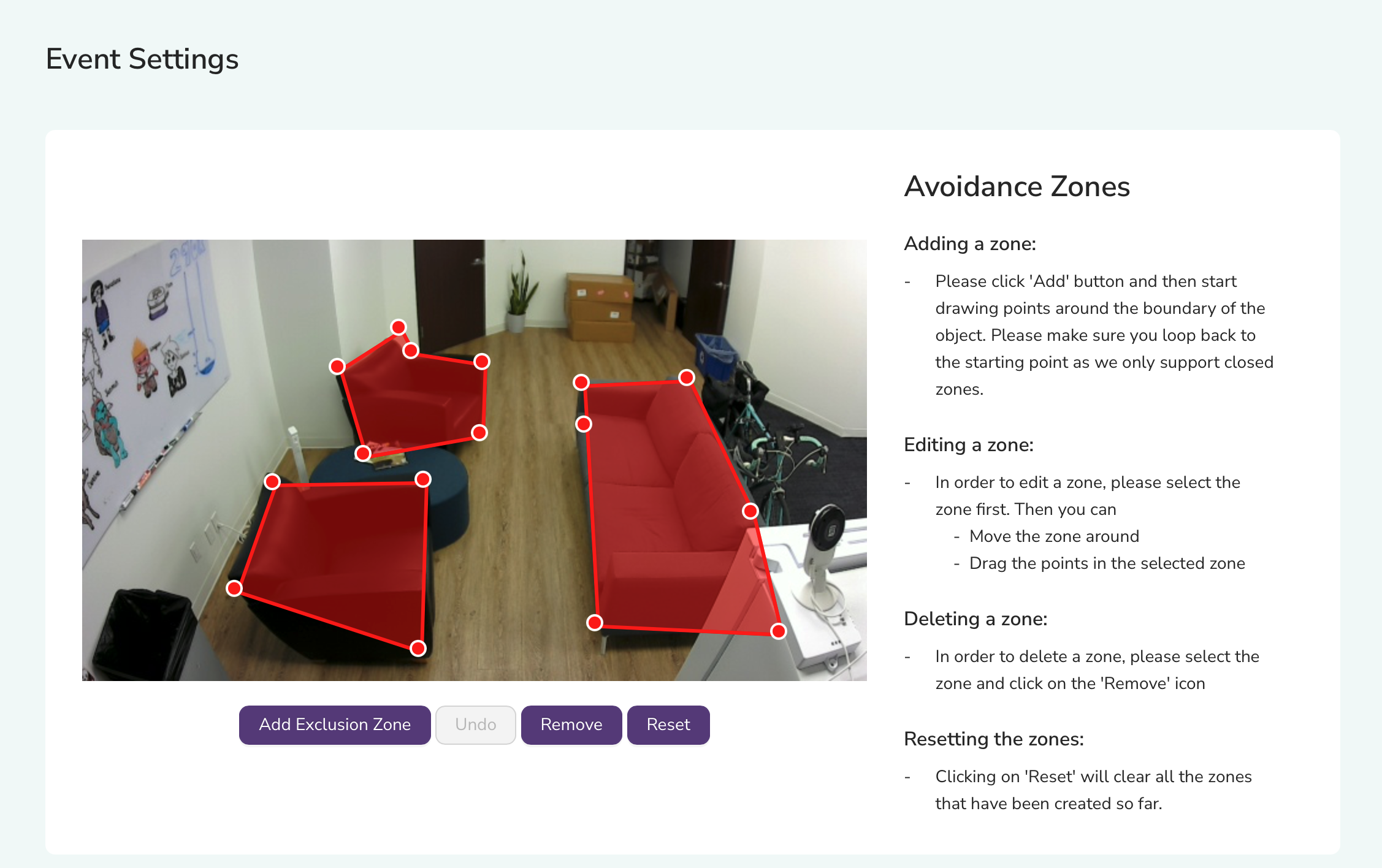
Setting Up Zones for Fall Detection and Bed Exit with KamiCare
Introduction Ensuring the safety and well-being of your loved ones is paramount, especially for seniors residing ...
Read More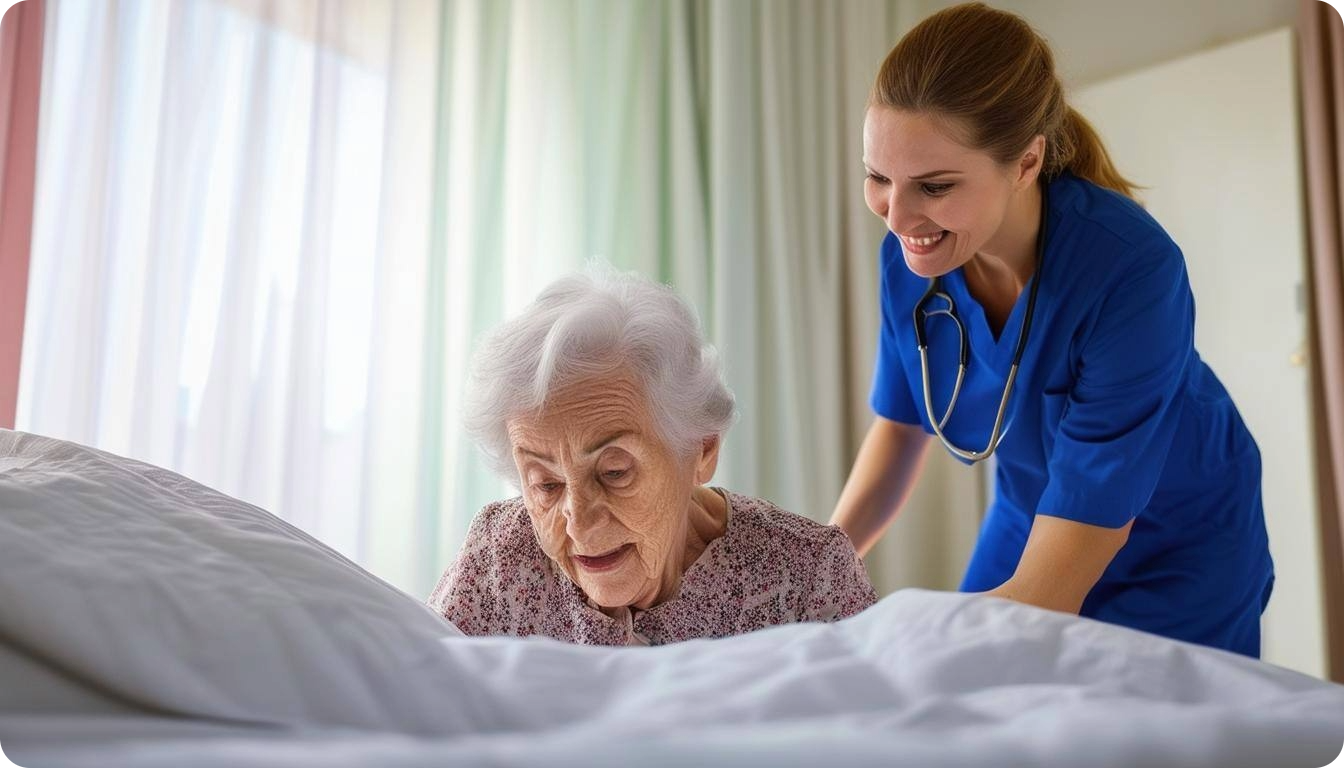
KamiCare Bed Exit Detection Enhances Resident Safety in Memory Care Communities
KamiCare Bed Exit Detection: Enabling A New Level of Proactive, Quality Care Bed Exit Detection is a smart feature ...
Read More